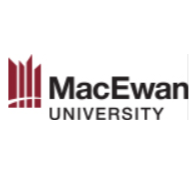
Level UP-Clustering of Covid data (part 1 of 2)
positions available: 1 The students will clean the Covid data collected from OUR WORLD in DATA and implement a clustering algorithm for Covid data in R based on existing libraries.
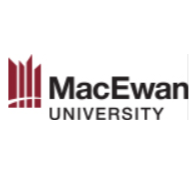
Level UP-Clustering of Covid data (part 2 of 2)
positions available: 1 The student will apply decision trees to explain the COVID data clustering. The student will interpret the results and write a report.
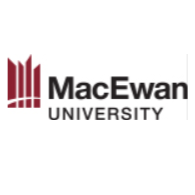
Level UP-Analyzing factors that cause Car speeding Phase 1
positions available: 2 This analysis used data from speed zones and traffic signs using methods such as functional data clustering algorithms. In this project, we will 1. Determine indicators associated with a car speeding such as known police zones, how enforcement is done in these areas and unknown information about the day (weather, holiday, sporting events) that may or may not affect the data. 2. Apply functional data clustering and model-based clustering algorithms
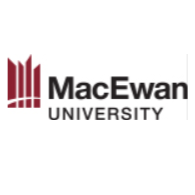
Level UP-Analyzing factors that cause Car speeding Phase 2
positions available: 2 This analysis used data from speed zones and traffic signs using methods such as functional data clustering algorithms. In this project, we will 1. Determine indicators associated with a car speeding such as known police zones, how enforcement is done in these areas and unknown information about the day (weather, holiday, sporting events) that may or may not affect the data. 2. Apply functional data clustering and model-based clustering algorithms
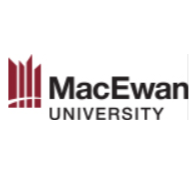
Level UP-Writing-testing a R package on clustering functional data (part 1 of 2)
positions available: 2 One student will write R/C code for the T-funHDDC algorithm for clustering functional data with outliers. The second student will do the testing.
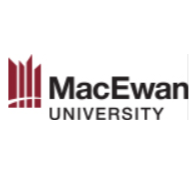
Level UP-Writing-testing a R package on clustering functional data (part 2 of 2)
positions available: 1 The student will write R/C code for the T-funHDDC algorithm for clustering functional data with outliers and the package manual.
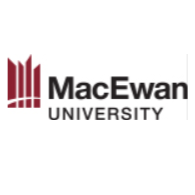
Developing CF for video data fit as multivariate functional data. Phase 1
positions available: 2 This project develops filters for functional data, specifically video data, that can be used to extend functional neural networks to convolutional neural networks. Filters work for data with relationships. These have been developed for photos and work for relationships in time series data. We plan to link these two filters into video data to filter unique properties of video (movement, colour change, and effects). To implement this project, we plan to use Python's functional data analysis package, pillow, and pandas. We will produce and distribute a package using GitHub so that interested teams can implement the filters. We will also produce this framework as there is no current format for turning videos into functional data.
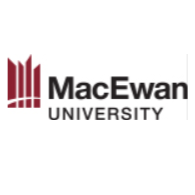
Developing CF for video data fit as multivariate functional data. Phase 2
positions available: 2 This project develops filters for functional data, specifically video data, that can be used to extend functional neural networks to convolutional neural networks. Filters work for data with relationships. These have been developed for photos and work for relationships in time series data. We plan to link these two filters into video data to filter unique properties of video (movement, colour change, and effects). To implement this project, we plan to use Python's functional data analysis package, pillow, and pandas. We will produce and distribute a package using GitHub so that interested teams can implement the filters. We will also produce this framework as there is no current format for turning videos into functional data.
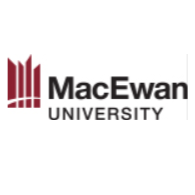
Developing CF for video data fit as multivariate functional data. Phase 3
positions available: 2 This project develops filters for functional data, specifically video data, that can be used to extend functional neural networks to convolutional neural networks. Filters work for data with relationships. These have been developed for photos and work for relationships in time series data. We plan to link these two filters into video data to filter unique properties of video (movement, colour change, and effects). To implement this project, we plan to use Python's functional data analysis package, pillow, and pandas. We will produce and distribute a package using GitHub so that interested teams can implement the filters. We will also produce this framework as there is no current format for turning videos into functional data.